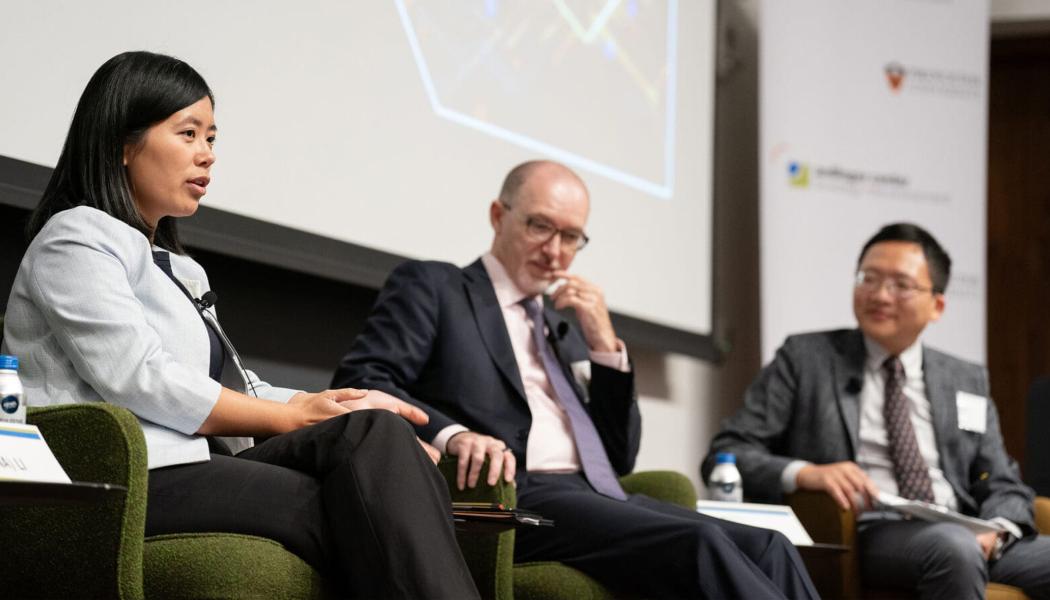
Left to right: Na (Lina) Li, Tassos Golnas and moderator Minjie Chen, an associate professor of electrical and computer engineering and the Andlinger Center for Energy and the Environment, discuss how AI can help to automate power grid operation during the “AI for Power” panel.
The rise of artificial intelligence (AI) poses both opportunities and risks for the clean energy transition, speakers agreed at the Andlinger Center for Energy and the Environment’s 13th annual meeting.
Titled “Energy for AI and AI for Energy,” the Oct. 29 conference convened experts from industry, academia and the public sector to explore the double-edged sword of AI. On one hand, the energy consumption from AI and its associated data centers will exacerbate challenges to already struggling clean energy ambitions. On the other, AI’s ability to quickly process and react to unprecedented amounts of data will unlock new ways of approaching energy and climate challenges, allowing people to access information and complete tasks at speeds previously seen as impossible.
“AI is influencing nearly every academic discipline — frankly, nearly every human endeavor,” said Jennifer Rexford, Princeton’s provost and the Gordon Y. S. Wu Professor in Engineering, during the day’s welcoming remarks. “This event is part of the conversation about how Princeton is advancing AI research to solve major societal challenges, including climate change.”
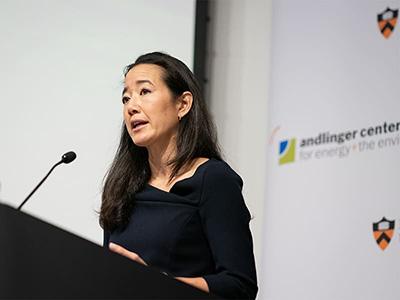
Setting the stage for the day’s discussions in her keynote address, Melanie Nakagawa, Microsoft’s chief sustainability officer, said the key to navigating the energy and environmental challenges of AI’s rise is to view it holistically, considering its full lifecycle impacts across an interconnected global ecosystem.
Behind each AI-powered tool is a piece of computing equipment in a physical data center, located within or near a community where people live and work. As AI continues to surge in popularity, Nakagawa said, so will the size and number of such data centers, which are often built from carbon-intensive materials like concrete and steel and have their own heating and cooling costs. She said 96% of Microsoft’s reported carbon emissions were from indirect emissions, including those embodied in the materials used to build and support these AI data centers.
“We want to operate as a good neighbor to these communities,” Nakagawa said.
To Nakagawa, being a good neighbor not only means listening and responding to the needs of communities in which Microsoft operates but also working with others to pool demand for cleaner building materials and advanced energy technologies. She pointed to a partnership between Microsoft, Google and steel manufacturer Nucor to aggregate demand and develop new business models for clean energy technologies to catalyze the energy transition worldwide.
“Ultimately, this is a systems challenge,” Nakagawa said. “We want to create an impact beyond our company, so we are investing in solutions and advocating for policies that can support a net-zero future for everyone.”
AI: One component of growing demand
Echoing Nakagawa’s systems approach, panelists underscored that AI and its associated data centers will be just one driver of future energy demand, alongside the wider adoption of electric vehicles and the growth of emerging energy technologies such as hydrogen electrolysis. Beyond AI, they said the real challenge is preparing the energy system for this period of sustained growth after decades of plateaued energy demand.
“The interconnection processes weren’t designed for anything other than central station dispatchable energy — most recently, combined cycle natural gas plants,” said Allison Clements, a former commissioner at the Federal Energy Regulatory Commission (FERC), which regulates the interstate transmission of energy resources. “We’ve got a situation now where there are new renewables projects that want to sign up, but they can’t get on.”
Clements said that regulatory processes need to evolve alongside technologies to pave the way for greater transmission capacity, which will be critical for allowing clean energy supply to keep pace with demand.
Matt DeNichilo, a partner at ECP, also said the unique characteristics of data centers — big, always-on energy consumers — mean that solar and wind renewables will need to be complemented with significant amounts of long-duration energy storage or dispatchable clean energy sources to ensure these data centers can operate sustainably.
In this way, Lucia Tian, head of clean energy and decarbonization technologies at Google, argued that AI’s growing energy footprint is an opportunity to grow global investments in clean energy technologies that will catalyze the broader energy transition.
Beyond accelerating the deployment of renewables like wind and solar, Tian pointed to Google’s recent investments into enhanced geothermal energy, advanced small modular nuclear reactors and long-duration energy storage, all of which could help Google meet its goal of achieving 24/7 carbon-free energy.
“Some of our early investments and partnerships can bring these emerging technologies that are at a premium today down the cost curve, so they can be available for everyone,” Tian said.
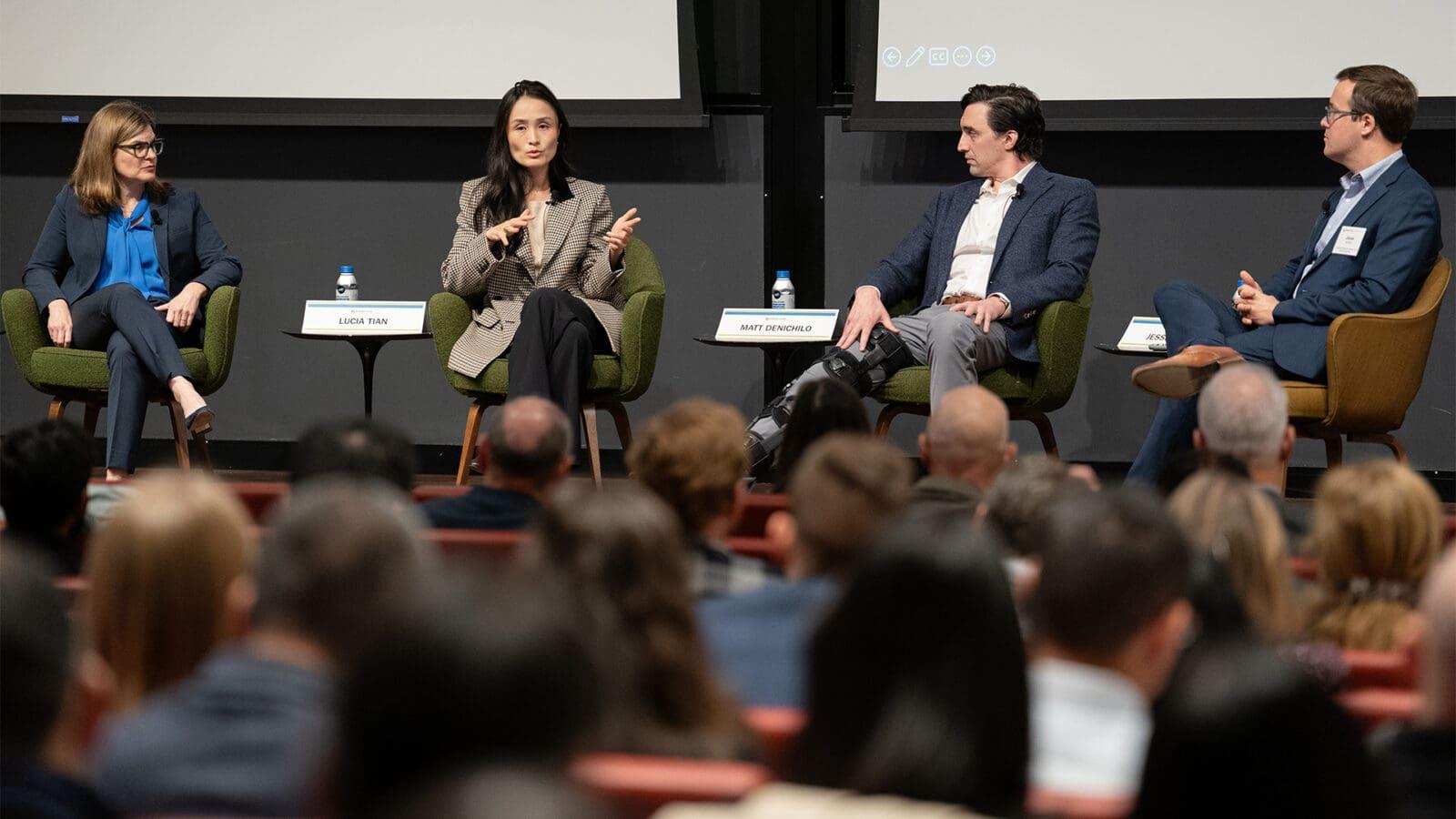
Pushing the limits of computing
Zooming in to consider challenges at the chip level, speakers on another panel discussed ongoing efforts to balance computing performance with AI’s voracious energy consumption.
For decades, engineers have managed to increase the performance of computing chips while minimizing costs. Recently, however, continued performance improvements have become increasingly difficult and more costly to make, resulting instead in the rapid buildout of data centers to meet the computing needs of AI.
“There is an insatiable demand for compute, and energy is really the limiting factor at every level, from the die to the module to the rack to the data center,” said Tom Gray, senior director of circuits research at Nvidia.
Despite increasing headwinds, Gray estimated that chip performance has risen nearly 1,000-fold over the past eight years. Most of those gains, Gray said, are from simpler number representation in chips and the optimization of computing networks themselves. However, Gray said these performance boosts have come astride a tripling in energy consumption, foreshadowing the challenges that the energy sector will face as demand for AI grows.
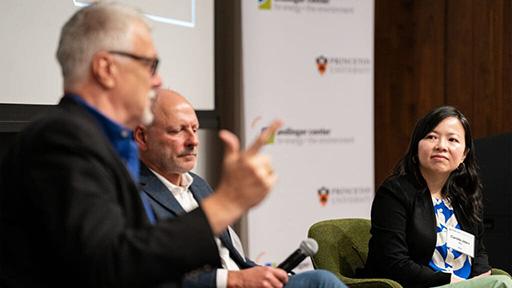
Mark Johnson, director of the Center for Advanced Manufacturing and professor of materials science and engineering at Clemson University, cautioned that without a careful approach, the rising energy demand from AI and the information sector will spur the lock-in of additional fossil fuel infrastructure, especially since the use of AI is still in its early stages.
Still, Stephen Kosonocky, a senior fellow at AMD, expressed optimism at the industry’s ability to overcome today’s computing bottlenecks, citing examples of how researchers overcame previous, seemingly insurmountable challenges through the development of new technological paradigms for computing.
“The power demand of computing and data centers isn’t going away, and conventional techniques for boosting efficiency are quickly running out of steam,” Kosonocky said. “What we need are new trajectories and new computing architectures to support greater efficiencies as AI evolves.”
While other panelists focused on the operational energy consumption of AI and data centers, panelist Carole-Jean Wu, a director of AI research at Meta, also highlighted that the embodied emissions from chip manufacturing — the carbon emissions generated from constructing each component — will contribute significantly to AI’s climate impacts.
“Operational emissions are only part of the total lifecycle emissions,” Wu said. “Going forward, we will see that the embodied emissions in the devices themselves will dominate.”
AI’s role in powering energy solutions
Despite its high energy consumption, speakers suggested that AI itself can be a tool for unlocking innovations that could accelerate the energy transition. They overviewed how AI is already helping to speed up problem-solving across technologies.
“At some level, whenever we say AI, we’re talking about optimization or applying a numerical understanding of a situation to gain a better outcome,” said Chris White, president of NEC Laboratories America. “And I can guarantee that we’re not going to run out of problems that need to be optimized.”
For instance, Egemen Kolemen, an associate professor of mechanical and aerospace engineering and the Andlinger Center for Energy and the Environment, as well as staff research physicist at the Princeton Plasma Physics Laboratory (PPPL), discussed how AI and machine learning tools can be applied to fusion energy, from enabling more robust diagnostics to providing real-time control for fusion reactions.
Na (Lina) Li, the Winokur Family Professor of Electrical Engineering and Applied Mathematics at Harvard University, talked about how reinforcement learning models could be deployed to automate aspects of power grid operations.
Yet Li also overviewed the challenges remaining for AI research before it can be applied to critical systems like the power grid, including whether the reinforcement learning process and learned control policies are safe and robust to uncertainty, whether the models can be scaled to large systems, and whether there is enough high-quality data available for training.
Tassos Golnas, a technology manager at the U.S. Department of Energy’s Solar Energy Technologies Office, discussed a proposed initiative from the Department of Energy that would address some of Li’s concerns, known as Frontiers in Artificial Intelligence for Science, Security and Technology (FASST). The initiative aims to build integrated AI systems by compiling high-quality and AI-ready data, scaling up supercomputing platforms and algorithms, and training and validating advanced AI models to accelerate innovations in topics from energy storage to fusion energy.
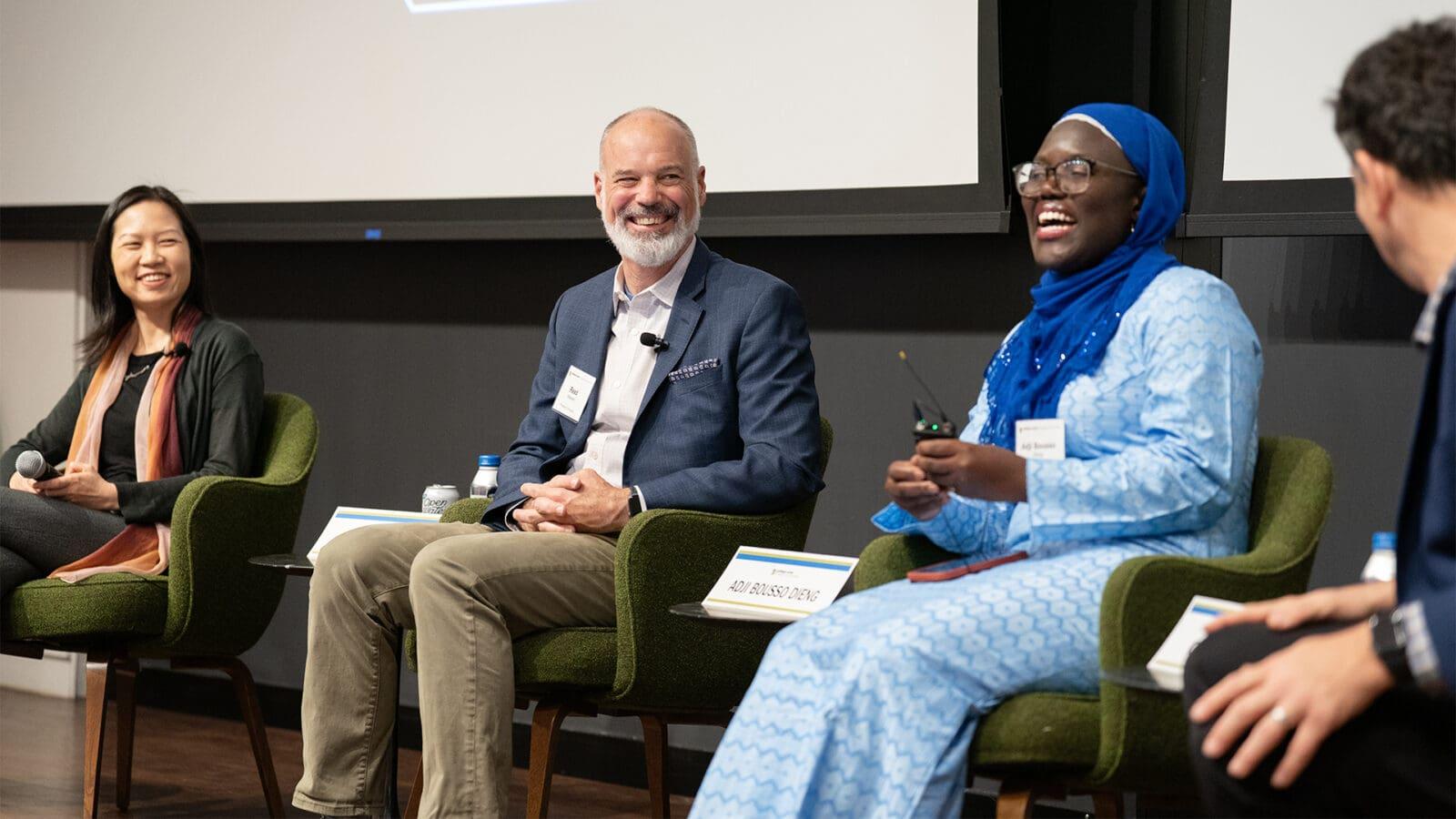
Thinking critically about AI in climate research
Speakers rounded out the day by expanding on the promise and pitfalls of AI and machine learning for climate and environmental science research.
Reed Maxwell, the William and Edna Macaleer Professor of Engineering and Applied Science, demonstrated that AI could accurately emulate a physics-based simulation of North American water table depths while producing results 1,000 times faster. This speed could boost these models’ relevance to water managers and decision-makers, whereas previous approaches could take days or weeks to yield results.
At the same time, speakers cautioned against AI becoming the knee-jerk approach to solving every problem. Adji Bousso Dieng, an assistant professor of computer science, said generative AI models often only capture certain aspects of a dataset. When used for materials discovery, for instance, Dieng said AI-driven molecular simulations often become stuck exploring one type of configuration rather than the entire design space.
As such, the panelists agreed that AI and machine learning are good complements to — but not replacements for — a physics-based understanding of earth systems.
“I always ask my students to start with simple models or to tell me why we should be using something more complicated,” said panelist Ning Lin, a professor of civil and environmental engineering who has used AI and machine learning to upscale hurricane hazard models. “Unless the more complicated model produces much better results, the simpler models are easier to understand and interpret.”
Ultimately, the panelists agreed the rise of AI holds great potential for enabling new, often multi- and interdisciplinary collaborations. That sentiment was echoed by Iain McCulloch, director of the Andlinger Center and the Gerhard R. Andlinger Professor in Energy and the Environment, in his remarks.
“The challenge we face is how best to leverage AI’s technological promises while mitigating its energy and environmental impacts,” said McCulloch. “And as we rise to meet that challenge, it is critical that we cultivate dialogue and build relationships across sectors, broadening our perspective on energy and environmental issues and collaborating on the solutions with the greatest impact.”
The article originally appeared on the Princeton Engineering website.